Topological analysis of multiple tables
Abstract
Keywords
Full Text:
PDFReferences
1. Dazy F, Le Barzic JF, Saporta G, et al. Evolutionary Data Analysis—Methods and Applications (French). Editions TECHNIP; 1996.
2. Escofier B, Pagès J. Implementation of MFA for Numerical, Qualitative, or Mixed Tables (French). Publication Interne de l’IRISA; 1985. p. 429.
3. Lavit C. Joint Analysis of Quantitative Tables (French). Editions Masson; 1988.
4. des Planttes HLH. Structuring Tables with Three Statistical Indices (French). Université des Sciences et Techniques du Languedoc; 1976.
5. Bouroche JM. Analysis of Ternary Data: Double Principal Component Analysis (French) [PhD thesis]. Université de Paris VI; 1975.
6. Abdesselam R. A topological clustering of individuals. In: Brito P, Dias JG, Lausen B, et al. Classification and Data Science in the Digital Age. Springer; 2022. pp. 1–8.
7. Abdesselam R. A Topological Clustering of Variables. Journal of Mathematics and System Science. 2021, 11(2): 1–17. doi: 10.17265/2159-5291/2021.02.001
8. Aljarah I, Faris H, Mirjalili S, et al. Evolutionary Data Clustering: Algorithms and Applications. Springer Singapore, 2021. doi: 10.1007/978-981-33-4191-3
9. Panagopoulos D. Topological data analysis and clustering. In: Daras NJ, Pardalos PM, Rassias M (editors). Analysis, Cryptography and Information Science. World Scientific; 2023.
10. Batagelj V, Bren M. Comparing resemblance measures. Journal of Classification. 1995, 12(1): 73-90. doi: 10.1007/bf01202268
11. Lesot MJ, Rifqi M, Benhadda H. Similarity measures for binary and numerical data: a survey. International Journal of Knowledge Engineering and Soft Data Paradigms. 2009, 1(1): 63. doi: 10.1504/ijkesdp.2009.021985
12. Zighed DA, Abdesselam R, Hadgu A. Topological Comparisons of Proximity Measures. Lecture Notes in Computer Science. Published online 2012: 379-391. doi: 10.1007/978-3-642-30217-6_32
13. Kim JH, Lee S. Tail bound for the minimal spanning tree of a complete graph. Statistics & Probability Letters. 2003, 64(4): 425-430. doi: 10.1016/s0167-7152(03)00208-6
14. Park JC, Shin H, Choi BK. Elliptic Gabriel graph for finding neighbors in a point set and its application to normal vector estimation. Computer-Aided Design. 2006, 38(6): 619-626. doi: 10.1016/j.cad.2006.02.008
15. Toussaint GT. The relative neighbourhood graph of a finite planar set. Pattern Recognition. 1980, 12(4): 261-268. doi: 10.1016/0031-3203(80)90066-7
16. Abdesselam R. Mixed principal component analysis (French). In: Classification: Crossed Points of View (French). Cépaduès; 2008. pp. 31–41.
17. Caillez F, Pagès JP. Introduction to Data Analysis (French). SMASH; 1976.
18. Lebart L. Survey data processing strategy (French). La Revue de MODULAD. 1989; 3: 21–29.
19. Ward JH. Hierarchical Grouping to Optimize an Objective Function. Journal of the American Statistical Association. 1963, 58(301): 236-244. doi: 10.1080/01621459.1963.10500845
20. Fowlkes EB, Mallows CL. A Method for Comparing Two Hierarchical Clusterings. Journal of the American Statistical Association. 1983, 78(383): 553-569. doi: 10.1080/01621459.1983.10478008
21. Hubert L, Arabie P. Comparing partitions. Journal of Classification. 1985, 2(1): 193-218. doi: 10.1007/bf01908075
22. 2021 economic reports of French regions (French). Available online: https://www.insee.fr/fr/information/6456000 (accessed on 31 December 2021).
23. Overview of renewable electricity (French). Available online: https://assets.rte-france.com/prod/public/2022-02/Pano-2021-T4.pdf (accessed on 31 December 2021).
24. Poverty in the regions (French). Available online: https://www.inegalites.fr/La-pauvrete-dans-les-regions (accessed on 31 December 2021).
25. France map of carbon footprint by region (French). Available online: https://www.hellocarbo.com/empreinte-carbone-francais-2021-par-region/ (accessed on 31 December 2021).
DOI: https://doi.org/10.59400/jam.v2i1.424
(60 Abstract Views, 33 PDF Downloads)
Refbacks
- There are currently no refbacks.
Copyright (c) 2024 Rafik Abdesselam

This work is licensed under a Creative Commons Attribution 4.0 International License.
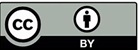
This site is licensed under a Creative Commons Attribution 4.0 International License.