Developing data science for structural safety analysis and pre-warning in civil engineering
Abstract
The development of data science in civil engineering has benefited from the rapid advances in sensor technology as well as data acquisition and storage. In contrast to traditional analysis and evaluation based on periodic inspection and full-scale test, the structural safety analysis and pre-warning can be achieved directly through the analysis of the design data of the newly built bridge and the monitoring data & test data of the bridge in service. Specifically, structural geometric data (length and cross-sectional area etc.), physical response data like displacement and stress, and vibration response data, such as acceleration and frequency, as well as the influence of the environment, e.g., temperature and humidity, must all be taken into account. Furthermore, the different sensitivity of different response data, which in turn affects structural safety analysis and pre-warning accuracy, is one of the current frontier sciences, i.e., the problem of multi-source (different response) data. It is expected that the development of data science will have very important theoretical research value and engineering practice significance for safety analysis and pre-warning in civil engineering, and is expected to bring new prospects for academia and industry.
Keywords
Full Text:
PDFReferences
1. Jin QW, Liu Z, Bin JC, Ren WX. Predictive analytic of in-service bridge structural performance from SHM data mining perspective: A case study. Shock and Vibration 2019. doi: 10.1155/2019/6847053
2. Sun FZ, Liu Y, Wang JX, Sun H. Symbolic physics learner: Discovering governing equations via Monte Carlo tree search. In: Proceedings of the 11th International Conference on Learning Representations (ICLR'2023); 1–5 May 2023; Kigali, Rwanda.
3. Jin QW, He SH, Liu Z. Non-vibration data-driven inverse evaluation analysis of in-service prefabricated slab-on-girder bridge. Innovative Infrastructure Solutions 2022; 42(7): 1–9. doi: 10.1007/s41062-021-00642-6
4. Shirota Y, Sari RF, Presekal A, Hashimoto T. Visualization of time series data change by statistical shape analysis. In: Proceedings of the 16th International Conferenceon Qualityin Research (QIR): International Symposium on Electrical and Computer Engineering; 22–24 July 2019; Padang, Indonesia.
5. Liu Z, Blasch E, Liao M, et al. Digital twin for predictive maintenance. In: Proceedings of the NDE 4.0, Predictive Maintenance, Communication, and Energy Systems: The Digital Transformation of NDE; 14–15 March 2023; California, United States.
6. Jin QW. Application Study on Bridge Structural Health Monitoring with Consideration of Structural Robustness Analysis Energy Method and Data Mining Technology [PhD thesis]. Hefei University of Technology; 2019.
(35 Abstract Views, 32 PDF Downloads)
Refbacks
- There are currently no refbacks.
Copyright (c) 2023 Qiwen Jin, Shuanhai He, Zheng Liu
License URL: http://creativecommons.org/licenses/by/4.0/
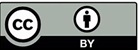
This site is licensed under a Creative Commons Attribution 4.0 International License.