Improving Leasing Viability: A comprehensive analysis of customer credibility checks and trustworthiness
Abstract
Keywords
Full Text:
PDFReferences
1. Durand D. Risk Elements in Consumer Instalment Financing. National Bureau of Economic Research; 1941.
2. Altman EI. Financial ratios, discriminant analysis and the prediction of corporate bankruptcy. The Journal of Finance 1968; 23(4): 589–609. doi: 10.1111/j.1540-6261.1968.tb00843.x
3. Krugman P. A model of balance-of-payments crises. Journal of Money, Credit and Banking 1979; 11(3): 311. doi: 10.2307/1991793
4. Richards VD, Laughlin EJ. A cash conversion cycle approach to liquidity analysis. Financial Management 1980; 9(1): 32. doi: 10.2307/3665310
5. Capon N. Credit scoring systems: A critical analysis. Journal of Marketing 1982; 46(2): 82–91. doi: 10.1177/002224298204600209
6. Emery GW. Measuring short-term liquidity. Journal of Cash Management 1984; 4(4): 25–32.
7. Viscione JA. Assessing financial distress. The Journal of Commercial Bank Lending Posted 1985; 39–55.
8. Gaharan CI. A Comparison of the Operating Funds Flow Measures of Cash, Net Quick Assets, and Working Capital in Predicting Future Cash Flow [PhD thesis]. Louisiana State University: 1988.
9. Arnold AJ, Clubb CDB, Manson S, et al. The relationship between earnings, funds flows and cash flows: Evidence for the UK. Accounting and Business Research 1991; 22(85): 13–19. doi: 10.1080/00014788.1991.9729413
10. Soenen LA. Cash conversion cycle and corporate profitability. Journal of cash Management 1993; 13: 53–53.
11. Crook J. Credit scoring an overview. Journal of Financial Services Marketing 1997; 2: 152–174.
12. Gallinger G. The current and quick ratios: Do they stand up to scrutiny? Business Credit 1997; 99(5): 24–25.
13. Schneider M, Tornell A. Balance sheet effects, bailout guarantees and financial crises. NBER Working Paper 8060. National Bureau of Economic Research; 2000.
14. Anderson R. The Credit Scoring Toolkit: Theory and Practice for Retail Credit Risk Management and Decision Automation. Oxford University Press; 2007. doi: 10.1093/oso/9780199226405.001.0001
15. Abdou H, Pointon J, El-Masry A. Neural nets versus conventional techniques in credit scoring in Egyptian banking. Expert Systems with Applications 2008; 35(3): 1275–1292. doi: 10.1016/j.eswa.2007.08.030
16. GST reforms and intergovernmental consideration in India. Asia Research Centre Working Paper 26. Asia Research Centre Working Paper; 2009.
17. Rao RK, Chakraborty P. Goods and services tax in India: An assessment of the base. Economic and Political Weekly 2010; 45(1): 49–54.
18. Abdou HA, Pointon J. Credit scoring, statistical techniques and evaluation criteria: A review of the literature. Intelligent Systems in Accounting, Finance and Management 2011; 18(2–3): 59–88. doi: 10.1002/isaf.325
19. Abuzayed B. Working capital management and firms’ performance in emerging markets: The case of Jordan. International Journal of Managerial Finance 2012; 8(2): 155–179. doi: 10.1108/17439131211216620
20. Blanco A, Pino-Mejías R, Lara J, et al. Credit scoring models for the microfinance industry using neural networks: Evidence from Peru. Expert Systems with Applications 2013; 40(1): 356–364. doi: 10.1016/j.eswa.2012.07.051
21. Guo G, Zhu F, Chen E, et al. From footprint to evidence. ACM Transactions on the Web 2016; 10(4): 1–38. doi: 10.1145/2996465
22. Bumacov V, Ashta A, Singh P. Credit scoring: A historic recurrence in microfinance. Strategic Change 2017; 26(6): 543–554. doi: 10.1002/jsc.2165
23. Bjorkegren D, Grissen D. Behavior revealed in mobile phone usage predicts loan repayment. SSRN Electronic Journal 2018. doi: 10.2139/ssrn.2611775
24. Hill J. Fintech and the Remaking of Financial Institutions. Academic Press; 2018. doi: 10.1016/c2016-0-03863-9
25. Shash AA, Qarra AA. Cash flow management of construction projects in Saudi Arabia. Project Management Journal 2018; 49(5): 48–63. doi: 10.1177/8756972818787976
DOI: https://doi.org/10.59400/fefs.v1i1.349
(87 Abstract Views, 21 PDF Downloads)
Refbacks
- There are currently no refbacks.
Copyright (c) 2024 Khushi Manjunath, Sundar Srinivasan, Praveen George
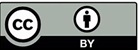
This site is licensed under a Creative Commons Attribution 4.0 International License.