Application of machine vision in drying process modeling of carrot slices
Abstract
Keywords
Full Text:
PDFReferences
1. Food and Agriculture Organization of the United Nations Carrots and Turnips, 2020. Available online: http: //www.fao.org/faostat/en/#data/QC/visualize. (Accessed on 10 October 2022).
2. Ahmad T, Cawood M, Iqbal Q, et al. Phytochemicals in Daucus carota and Their Health Benefits. Foods. 2019; 8(9): 424. doi: 10.3390/foods8090424
3. Dhalsamant K, Tripathy PP, Shrivastava SL. Effect of pretreatment on rehydration, colour and nanoindentation properties of potato cylinders dried using a mixed‐mode solar dryer. Journal of the Science of Food and Agriculture. 2017; 97(10): 3312–3322. doi: 10.1002/jsfa.8181
4. Wang H, Fang XM, Sutar PP, et al. Effects of vacuum-steam pulsed blanching on drying kinetics, colour, phytochemical contents, antioxidant capacity of carrot and the mechanism of carrot quality changes revealed by texture, microstructure and ultrastructure. Food Chemistry. 2021; 338: 127799. doi: 10.1016/j.foodchem.2020.127799
5. Sharma S, Dhalsamant K, Tripathy PP. Application of computer vision technique for physical quality monitoring of turmeric slices during direct solar drying. Journal of Food Measurement and Characterization. 2018; 13(1): 545-558. doi: 10.1007/s11694-018-9968-0
6. Sharma S, Dhalsamant K, Tripathy PP, et al. Quality analysis and drying characteristics of turmeric (Curcuma longa L.) dried by hot air and direct solar dryers. LWT. 2021; 138: 110687. doi: 10.1016/j.lwt.2020.110687
7. Mudahar GS, Toledo RT, Floros JD, et al. Optimization of Carrot Dehydration Process using Response Surface Methodology. Journal of Food Science. 1989; 54(3): 714-719. doi: 10.1111/j.1365-2621.1989.tb04688.x
8. Dey D, Dhalsamant K, Tripathy PP. Effect of different pre-treatments on rehydration kinetics of solar and hot-air dried Fuji apple slices. Trends in Horticulture. 2023; 6(2): 3185. doi: 10.24294/th.v6i2.3185
9. Kakani V, Nguyen VH, Kumar BP, et al. A critical review on computer vision and artificial intelligence in food industry. Journal of Agriculture and Food Research. 2020; 2: 100033. doi: 10.1016/j.jafr.2020.100033
10. Jahanbakhshi A, Kheiralipour K. Evaluation of image processing technique and discriminant analysis methods in postharvest processing of carrot fruit. Food Science & Nutrition. 2020; 8(7): 3346-3352. doi: 10.1002/fsn3.1614
11. Tian H, Wang T, Liu Y, et al. Computer vision technology in agricultural automation —A review. Information Processing in Agriculture. 2020; 7(1): 1-19. doi: 10.1016/j.inpa.2019.09.006
12. Zhu H, Yang L, Sun Y, et al. Identifying carrot appearance quality by an improved dense CapNet. Journal of Food Process Engineering. 2020; 44(1). doi: 10.1111/jfpe.13586
13. Mohebbi M, Akbarzadeh-T MR, Shahidi F, et al. Computer vision systems (CVS) for moisture content estimation in dehydrated shrimp. Computers and Electronics in Agriculture. 2009; 69(2): 128-134. doi: 10.1016/j.compag.2009.07.005
14. Aghbashlo M, Hosseinpour S, Mujumdar AS. Application of Artificial Neural Networks (ANNs) in Drying Technology: A Comprehensive Review. Drying Technology. 2015; 33(12): 1397-1462. doi: 10.1080/07373937.2015.1036288
15. El-Mesery H, Mao H, Abomohra A. Applications of Non-destructive Technologies for Agricultural and Food Products Quality Inspection. Sensors. 2019; 19(4): 846. doi: 10.3390/s19040846
16. Ebrahimi E, Mollazade K, Babaei S. Toward an automatic wheat purity measuring device: A machine vision-based neural networks-assisted imperialist competitive algorithm approach. Measurement. 2014; 55: 196-205. doi: 10.1016/j.measurement.2014.05.003
17. Sampson DJ, Chang YK, Rupasinghe HPV, et al. A dual-view computer-vision system for volume and image texture analysis in multiple apple slices drying. Journal of Food Engineering. 2014; 127: 49-57. doi: 10.1016/j.jfoodeng.2013.11.016
18. Majumdar S, Jayas DS. Classification of cereal grains using machine vision: IV. Combined morphology, color, and texture models. Transactions of the ASAE. 2000; 43(6): 1689-1694. doi: 10.13031/2013.3069
19. Zhang Y, Wang S, Ji G, et al. Fruit classification using computer vision and feedforward neural network. Journal of Food Engineering. 2014; 143: 167-177. doi: 10.1016/j.jfoodeng.2014.07.001
20. Fathi M, Mohebbi M, Razavi SMA. Application of Image Analysis and Artificial Neural Network to Predict Mass Transfer Kinetics and Color Changes of Osmotically Dehydrated Kiwifruit. Food and Bioprocess Technology. 2009; 4(8): 1357-1366. doi: 10.1007/s11947-009-0222-y
21. Hosseinpour S, Rafiee S, Mohtasebi SS. Application of Image Processing to Analyze Shrinkage and Shape Changes of Shrimp Batch during Drying. Drying Technology. 2011; 29(12): 1416-1438. doi: 10.1080/07373937.2011.587620
22. Hosseinpour S, Rafiee S, Mohtasebi SS, et al. Application of computer vision technique for on-line monitoring of shrimp color changes during drying. Journal of Food Engineering. 2013; 115(1): 99-114. doi: 10.1016/j.jfoodeng.2012.10.003
23. Zenoozian MS, Feng H, Razavi SMA, et al. Image analysis and dynamic modeling of thin-layer drying of osmotically dehydrated pumpkin. Journal of Food Processing and Preservation. 2008; 32(1): 88-102. doi: 10.1111/j.1745-4549.2007.00167.x
24. Sturm B, Hofacker WC, Hensel O. Optimizing the Drying Parameters for Hot-Air–Dried Apples. Drying Technology. 2012; 30(14): 1570-1582. doi: 10.1080/07373937.2012.698439
25. Jahns G, Nielsen HM, Paul W. Measuring image analysis attributes and modelling fuzzy consumer aspects for tomato quality grading. Comput. Electron. Agric. 2001; 31(1): 17-29. doi: 10.1016/S0168-1699(00)00171-X
26. Blaschke T. Object based image analysis for remote sensing. ISPRS Journal of Photogrammetry and Remote Sensing. 2010; 65(1): 2-16. doi: 10.1016/j.isprsjprs.2009.06.004
27. Behroozi Khazaei N, Tavakoli T, Ghassemian H, et al. Applied machine vision and artificial neural network for modeling and controlling of the grape drying process. Computers and Electronics in Agriculture. 2013; 98: 205-213. doi: 10.1016/j.compag.2013.08.010
28. Dhalsamant, K., Tripathy, P. P., & Shrivastava, S. L. (2017). Moisture transfer modeling during solar drying of potato cylinders considering shrinkage. International Journal of Green Energy, 14(2), 184-195. doi: 10.1080/15435075.2016.1256290
29. Hosseinpour S, Rafiee S, Aghbashlo M, et al. Computer Vision System (CVS) for In-Line Monitoring of Visual Texture Kinetics During Shrimp (Penaeus Spp.) Drying. Drying Technology. 2014; 33(2): 238-254. doi: 10.1080/07373937.2014.947513
30. Fernandez L, Castillero C, Aguilera JM. An application of image analysis to dehydration of apple discs. Journal of Food Engineering. 2005; 67(1-2): 185-193. doi: 10.1016/j.jfoodeng.2004.05.070
31. Poona NK, Ismail R. Using Boruta-Selected Spectroscopic Wavebands for the Asymptomatic Detection of Fusarium Circinatum Stress. IEEE Journal of Selected Topics in Applied Earth Observations and Remote Sensing. 2014; 7(9): 3764-3772. doi: 10.1109/jstars.2014.2329763
32. Lim DK, Long NP, Mo C, et al. Combination of mass spectrometry-based targeted lipidomics and supervised machine learning algorithms in detecting adulterated admixtures of white rice. Food Research International. 2017; 100: 814-821. doi: 10.1016/j.foodres.2017.08.006
33. Min W, Zhou P, Xu L, et al. From Plate to Production: Artificial Intelligence in Modern Consumer-Driven Food Systems. arXiv preprint arXiv:2311.02400
34. Banda T, Farid AA, Li C, et al. Application of machine vision for tool condition monitoring and tool performance optimization–a review. The International Journal of Advanced Manufacturing Technology. 2022; 121(11-12): 7057-7086. doi: 10.1007/s00170-022-09696-x
35. Zheng C, Sun DW, Zheng L. Recent applications of image texture for evaluation of food qualities—A review. Trends in Food Science & Technology. 2006; 17(3): 113-128. doi: 10.1016/j.tifs.2005.11.006
36. Gao X, Tan J. Analysis of expanded‐food texture by image processing part II: Mechanical properties. Journal of Food Process Engineering. 1996; 19(4): 445-456. doi: 10.1111/j.1745-4530.1996.tb00404.x
37. Brosnan T, Sun DW. Improving quality inspection of food products by computer vision—A review. J. Food Eng. 2004; 61: 3-16. doi: 10.1016/S0260-8774(03)00183-3
38. Haralick RM, Shanmugam K, Dinstein I. Textural Features for Image Classification. IEEE Transactions on Systems, Man, and Cybernetics. 1973; SMC-3(6): 610-621. doi: 10.1109/tsmc.1973.4309314
39. Kursa MB, Rudnicki WR. Feature Selection with theBorutaPackage. Journal of Statistical Software. 2010; 36(11). doi: 10.18637/jss.v036.i11
40. Chen J, Chia N, Kalari KR, et al. Multiple sclerosis patients have a distinct gut microbiota compared to healthy controls. Scientific Reports. 2016; 6(1). doi: 10.1038/srep28484
41. Chen CR, Ramaswamy HS, Alli I. Prediction of quality changes during osmo-convective drying of blueberries using neural network models for process optimization. Drying Technology. 2001; 19(3-4): 507-523. doi: 10.1081/drt-100103931
42. Cheng F, Chen FN, Ying YB. Image Recognition of Unsound Wheat Using Artificial Neural Network. 2010 Second WRI Global Congress on Intelligent Systems. Published online December 2010. doi: 10.1109/gcis.2010.220
43. Uhlig S, Colson B, Hettwer K, et al. Valid machine learning algorithms for multiparameter methods. Accreditation and Quality Assurance. 2019; 24(4): 271-279. doi: 10.1007/s00769-019-01384-w
44. Dhalsamant K. Development, validation, and comparison of FE modeling and ANN model for mixed‐mode solar drying of potato cylinders. Journal of Food Science. 2021; 86(8): 3384-3402. doi: 10.1111/1750-3841.15847
45. Dhalsamant K, Tripathy PP, Shrivastava SL. Effect of sodium metabisulfite pretreatment on micrographs, surface roughness and X-ray diffraction analyses of solar dried potato cylinders. Innovative Food Science & Emerging Technologies. 2018; 47: 399-411. doi: 10.1016/j.ifset.2018.03.014
46. Dhalsamant K, Tripathy PP, Shrivastava SL. Heat transfer analysis during mixed-mode solar drying of potato cylinders incorporating shrinkage: Numerical simulation and experimental validation. Food and Bioproducts Processing. 2018; 109: 107-121. doi: 10.1016/j.fbp.2018.03.005
47. Doymaz I. Drying kinetics, rehydration and colour characteristics of convective hot-air drying of carrot slices. Heat and Mass Transfer. 2016; 53(1): 25-35. doi: 10.1007/s00231-016-1791-8
48. Demiray E, Tulek Y. Color Degradation Kinetics of Carrot (Daucus carota L.) Slices during Hot Air Drying. Journal of Food Processing and Preservation. 2014; 39(6): 800-805. doi: 10.1111/jfpp.12290
(115 Abstract Views, 48 PDF Downloads)
Refbacks
- There are currently no refbacks.
Copyright (c) 2024 Gourab Basu, Kshanaprava Dhalsamant, Punyadarshini Punam Tripathy, Sonu Sharma

This work is licensed under a Creative Commons Attribution-NonCommercial 4.0 International License.
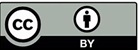
This site is licensed under a Creative Commons Attribution 4.0 International License.