Application of computer vision in livestock and crop production—A review
Abstract
Nowadays, it is a challenge for farmers to produce healthier food for the world population and save land resources. Recently, the integration of computer vision technology in field and crop production ushered in a new era of innovation and efficiency. Computer vision, a subfield of artificial intelligence, leverages image and video analysis to extract meaningful information from visual data. In agriculture, this technology is being utilized for tasks ranging from disease detection and yield prediction to animal health monitoring and quality control. By employing various imaging techniques, such as drones, satellites, and specialized cameras, computer vision systems are able to assess the health and growth of crops and livestock with unprecedented accuracy. The review is divided into two parts: Livestock and Crop Production giving the overview of the application of computer vision applications within agriculture, highlighting its role in optimizing farming practices and enhancing agricultural productivity.
Keywords
Full Text:
PDFReferences
1. Tassinari P, Bovo M, Benni S, et al. A computer vision approach based on deep learning for the detection of dairy cows in free stall barn. Computers and Electronics in Agriculture 2021; 182: 106030. doi: 10.1016/j.compag.2021.106030
2. Fernandes AFA, Dórea JRR, Rosa GJ de M. Image analysis and computer vision applications in animal sciences: An overview. Frontiers in Veterinary Science 2020; 7. doi: 10.3389/fvets.2020.551269
3. Pradana ZH, Hidayat B, Darana S. Beef cattle weight determine by using digital image processing. In: Proceedings of the 2016 International Conference on Control, Electronics, Renewable Energy and Communications (ICCEREC); 13–15 September 2016; Bandung, Indonesia. pp. 179–184 doi: 10.1109/iccerec.2016.7814955
4. Önder H, Ari A, Ocak S, et al. Use of image analysis in animal science. Journal of Information Technology in Agriculture 2020; 1.
5. Nasirahmadi A, Edwards SA, Sturm B. Implementation of machine vision for detecting behaviour of cattle and pigs. Livestock Science 2017; 202: 25–38. doi: 10.1016/j.livsci.2017.05.014
6. Eduardo FC, Marta MA, Benjamin I, et al. Motion-based video monitoring for early detection of livestock diseases: The case of African swine fever. PLoS One 2017; 12(9): e0183793. doi: 10.1371/journal.pone.0183793
7. Patrício DI, Chen C, Larsen MLV, Berckmans D. Review: Precision livestock farming: Building ‘digital representations’ to bring the animals closer to the farmer. Animal 2019; 13(12): 3009–3017. doi: 10.1017/S175173111900199X
8. Fernández-Carrión E, Martínez-Avilés M, Ivorra B, et al. Motion-based video monitoring for early detection of livestock diseases: The case of African swine fever. PLOS ONE 2017; 12(9): e0183793. doi: 10.1371/journal.pone.0183793
9. Patrício DI, Rieder R. Computer vision and artificial intelligence in precision agriculture for grain crops: A systematic review. Computers and Electronics in Agriculture 2018; 153: 69–81. doi: 10.1016/j.compag.2018.08.001
10. Mamai O, Parsova V, Lipatova N, et al. The system of effective management of crop production in modern conditions. BIO Web of Conferences 2020; 17: 00027. doi: 10.1051/bioconf/20201700027
11. Tian H, Wang T, Liu Y, et al. Computer vision technology in agricultural automation—A review. Information Processing in Agriculture 2019; 7(1): 1–19. doi: 10.1016/j.inpa.2019.09.006
12. Liakos K, Busato P, Moshou D, et al. Machine learning in agriculture: A review. Sensors 2018; 18(8): 2674. doi: 10.3390/s18082674
13. Tian H, Wang T, Liu Y, et al. Computer vision technology in agricultural automation—A review. Information Processing in Agriculture 2020; 7(1): 1–19. doi: 10.1016/j.inpa.2019.09.006
14. Redmond RS, Coenelia W, Ibrahim AH, et al. Research and development in agricultural robotics: A perspective of digital farming. International Journal of Agricultural and Biological Engineering 2018; 11(4): 1–14. doi: 10.25165/j.ijabe.20181104.4278
15. Foglia MM, Reina G. Agricultural robot for radicchio harvesting. Journal of Field Robotics 2006; 23(6–7): 363–377. doi: 10.1002/rob.20131
16. Ramin Shamshiri R, Weltzien C, et al. Research and development in agricultural robotics: A perspective of digital farming. International Journal of Agricultural and Biological Engineering 2018; 11(4): 1–11. doi: 10.25165/j.ijabe.20181104.4278
17. Vithu P, Moses JA. Machine vision system for food grain quality evaluation: A review. Trends in Food Science & Technology 2016; 56: 13–20. doi: 10.1016/j.tifs.2016.07.011
18. Wang S, Jiang H, Qiao Y, et al. The research progress of vision-based artificial intelligence in smart pig farming. Sensors 2022; 22(17): 6541. doi: 10.3390/s22176541
19. Diba A, Sharma V, Pazandeh A, et al. Weakly supervised cascaded convolutional networks. In: Proceedings of the 2017 IEEE Conference on Computer Vision and Pattern Recognition (CVPR); 21–26 July 2017; Honolulu, HI, USA. pp. 5131–5139. doi: 10.1109/CVPR.2017.545
20. Ronald T. Computer vision for smart farming and sustainable agriculture. In: Proceedings of the 2020 IST-Africa Conference (IST-Africa); 18–22 May 2020; Kampala, Uganda. pp. 1–8.
21. Binch A, Fox CW. Controlled comparison of machine vision algorithms for Rumex and Urtica detection in grassland. Computers and Electronics in Agriculture 2017; 140: 123–138. doi: 10.1016/j.compag.2017.05.018
22. Jose MR, Kaakinen H. An object detection application approach to analyze cattle under camera surveillance. Available online: https://centriabulletin.fi/object-detection/ (accessed on 26 January 2024).
23. Mediaan Conclusion. Using computer vision in the calving process. Available online: https://mediaan.com/mediaan-blog/computer-vision-calving (accessed on 26 January 2024).
24. Nye J, Zingaretti LM, Pérez-Enciso M. Estimating conformational traits in dairy cattle with DeepAPS: A two-step deep learning automated phenotyping and segmentation approach. Frontiers in Genetics 2020; 11. doi: 10.3389/fgene.2020.00513
25. Moore KL, Mrode R, Coffey MP. Genetic parameters of Visual Image Analysis primal cut carcass traits of commercial prime beef slaughter animals. Animal 2017; 11(10): 1653–1659. doi: 10.1017/s1751731117000489
26. Gupta H, Jindal P, Verma OP, et al. Computer vision-based approach for automatic detection of dairy cow breed. Electronics 2022; 11(22): 3791. doi: 10.3390/electronics11223791
27. Deng J, Dong W, Socher R, et al. ImageNet: A large-scale hierarchical image database. In: Proceedings of the 2009 IEEE Conference on Computer Vision and Pattern Recognition; 20–25 June 2009; Miami, FL, USA. pp. 248–255. doi: 10.1109/cvpr.2009.5206848
28. Everingham M, Van Gool L, Williams CKI, et al. The pascal visual object classes (VOC) challenge. International Journal of Computer Vision 2009; 88(2): 303–338. doi: 10.1007/s11263-009-0275-4
29. Lin TY, Maire M, Belongie S, et al. Microsoft COCO: Common objects in context. In: Fleet D, Pajdla T, Schiele B, Tuytelaars T (editors). Computer Vision—ECCV 2014. ECCV 2014. Lecture Notes in Computer Science. Springer, Cham; 2014. doi: 10.1007/978-3-319-10602-1_48
30. Bhole A, Udmale SS, Falzon O, et al. CORF3D contour maps with application to Holstein cattle recognition from RGB and thermal images. Expert Systems with Applications 2022; 192: 116354. doi: 10.1016/j.eswa.2021.116354
31. Andrew W, Greatwood C, Burghardt T. Visual localisation and individual identification of holstein friesian cattle via deep learning. In: Proceedings of the 2017 IEEE International Conference on Computer Vision Workshops (ICCVW); 22–29 October 2017; Venice, Italy. pp. 2850–2859. doi: 10.1109/ICCVW.2017.336
32. Andrew W, Greatwood C, Burghardt T. Aerial animal biometrics: Individual friesian cattle recovery and visual identification via an autonomous UAV with onboard deep inference. 2019 IEEE/RSJ International Conference on Intelligent Robots and Systems (IROS); 3–8 November 2019; Macau, China. pp. 237–243. doi: 10.1109/IROS40897.2019.8968555
33. Li G, Huang Y, Chen Z, et al. Practices and applications of convolutional neural network-based computer vision systems in animal farming: A review. Sensors 2021; 21(4): 1492. doi: 10.3390/s21041492
34. Arulmozhi E, Bhujel A, Moon BE, et al. The application of cameras in precision pig farming: An overview for swine-keeping professionals. Animals 2021; 11(8): 2343. doi: 10.3390/ani11082343
35. Albernaz-Gonçalves R, Olmos G, Hötzel MJ. My pigs are ok, why change?—Animal welfare accounts of pig farmers. Animal 2021; 15(3): 100154. doi: 10.1016/j.animal.2020.100154
36. Rauw WM, Rydhmer L, Kyriazakis I, et al. Prospects for sustainability of pig production in relation to climate change and novel feed resources. Journal of the Science of Food and Agriculture 2020; 100(9): 3575–3586. doi: 10.1002/jsfa.10338
37. Chijioke Ojukwu C, Feng Y, et al. Development of a computer vision system to detect inactivity in group-housed pigs. International Journal of Agricultural and Biological Engineering 2020; 13(1): 42–46. doi: 10.25165/j.ijabe.20201301.5030
38. Haladjian J, Ermis A, Hodaie Z, et al. iPig: Towards Tracking the Behavior of Free-roaming Pigs. Association for Computing Machinery; 2017. doi: 10.1145/3152130.3152145
39. Zhu W, Guo Y, Jiao P, et al. Recognition and drinking behaviour analysis of individual pigs based on machine vision. Livestock Science 2017; 205: 129–136. doi: 10.1016/j.livsci.2017.09.003
40. Shao H, Pu J, Mu J. Pig-posture recognition based on computer vision: Dataset and exploration. Animals 2021; 11(5): 1295. doi: 10.3390/ani11051295
41. Wongsriworaphon A, Arnonkijpanich B, Pathumnakul S. An approach based on digital image analysis to estimate the live weights of pigs in farm environments. Computers and Electronics in Agriculture 2015; 115: 26–33. doi: 10.1016/j.compag.2015.05.004
42. Li J, Green-Miller AR, Hu X, et al. Barriers to computer vision applications in pig production facilities. Computers and Electronics in Agriculture 2022; 200: 107227. doi: 10.1016/j.compag.2022.107227
43. Hendriks WH, Verstegen MWA, Babinszky L (editors). Poultry and Pig Nutrition: Challenges of the 21st Century. Wageningen Academic; 2019. doi: 10.3920/978-90-8686-884-1
44. Sun R, Zhang S, Wang T, et al. Willingness and influencing factors of pig farmers to adopt Internet of things technology in food traceability. Sustainability 2021; 13(16): 8861. doi: 10.3390/su13168861
45. Krizhevsky A, Sutskever I, Hinton GE. ImageNet classification with deep convolutional neural networks. Communications of the ACM 2017; 60(6): 84–90. doi: 10.1145/3065386
46. Chen LC, Papandreou G, Kokkinos I, et al. DeepLab: Semantic image segmentation with deep convolutional nets, atrous convolution, and fully connected CRFs. IEEE Transactions on Pattern Analysis and Machine Intelligence 2018; 40(4): 834–848. doi: 10.1109/tpami.2017.2699184
47. Szegedy C, Vanhoucke V, Ioffe S, et al. Rethinking the inception architecture for computer vision. In: Proceedings of the 2016 IEEE Conference on Computer Vision and Pattern Recognition (CVPR); 27–30 June 2016; Las Vegas, NV, USA. pp. 2818–2826, doi: 10.1109/CVPR.2016.308
48. Chen C, Zhu W, Steibel J, et al. Recognition of aggressive episodes of pigs based on convolutional neural network and long short-term memory. Computers and Electronics in Agriculture 2020; 169: 105166. doi: 10.1016/j.compag.2019.105166
49. Riekert M, Opderbeck S, Wild A, et al. Model selection for 24/7 pig position and posture detection by 2D camera imaging and deep learning. Computers and Electronics in Agriculture 2021; 187: 106213. doi: 10.1016/j.compag.2021.106213
50. Gan H, Li S, Ou M, et al. Fast and accurate detection of lactating sow nursing behavior with CNN-based optical flow and features. Computers and Electronics in Agriculture 2021; 189: 106384. doi: 10.1016/j.compag.2021.106384
51. Ji YP, Yang Y, Liu G. Recognition of pig eating and drinking behavior based on visible spectrum and YOLOv2. Spectroscopy and Spectral Analysis 2020; 40(05): 1588–1594. doi: 10.3964/j.issn.1000-0593(2020)05-1588-07
52. Neethirajan, S. Automated tracking systems for the assessment of farmed poultry. Animals 2022; 12: 232. doi: 10.3390/ani12030232
53. Guo Y, Chai L, Aggrey SE, et al. A machine vision-based method for monitoring broiler chicken floor distribution. Sensors 2020; 20(11): 3179. doi: 10.3390/s20113179
54. Jerine van der E, Guzhva O, Voss A, et al. Seeing is caring—Automated assessment of resource use of broilers with computer vision techniques. Frontiers in Animal Science 2022; 3. doi: 10.3389/fanim.2022.945534
55. Okinda C, Nyalala I, Korohou T, et al. A review on computer vision systems in monitoring of poultry: A welfare perspective. Artificial Intelligence in Agriculture 2020; 4: 184–208. doi: 10.1016/j.aiia.2020.09.002
56. Abd Aziz NSN, Mohd Daud S, Dziyauddin RA, et al. A review on computer vision technology for monitoring poultry farm—Application, hardware, and software. IEEE Access 2021; 9: 12431–12445. doi: 10.1109/access.2020.3047818
57. Mortensen AK, Lisouski P, Ahrendt P. Weight prediction of broiler chickens using 3D computer vision. Computers and Electronics in Agriculture 2016; 123: 319–326. doi: 10.1016/j.compag.2016.03.011
58. Aydin A. Using 3D vision camera system to automatically assess the level of inactivity in broiler chickens. Computers and Electronics in Agriculture 2017; 135: 4–10. doi: 10.1016/j.compag.2017.01.024
59. Zhuang X, Bi M, Guo J, et al. Development of an early warning algorithm to detect sick broilers. Computers and Electronics in Agriculture 2018; 144: 102–113. doi: 10.1016/j.compag.2017.11.032
60. Karthikeyan A, Siva M, Reshma A, et al. Applications of artificial intelligence in poultry industry. Available online: https://www.pashudhanpraharee.com/applications-of-artificial-intelligence-in-poultry-industry/ (accessed on 26 January 2024).
61. Dawkins MS, Wang L, Ellwood SA, et al. Optical flow, behaviour and broiler chicken welfare in the UK and Switzerland. Applied Animal Behaviour Science 2021; 234: 105180. doi: 10.1016/j.applanim.2020.105180
62. Wu D, Cui D, Zhou M, et al. Information perception in modern poultry farming: A review. Computers and Electronics in Agriculture 2022; 199: 107131. doi: 10.1016/j.compag.2022.107131
63. George AS, George ASH. Optimizing poultry production through advanced monitoring and control systems. Partners Universal International Innovation Journal 2023; 1(5): 77–97. doi: 10.5281/zenodo.10050352
64. Ammad-uddin M, Ayaz M, Aggoune EH, et al. Wireless sensor network: A complete solution for poultry farming. In: Proceedings of the 2014 IEEE 2nd International Symposium on Telecommunication Technologies (ISTT); 24–26 November 2014; Langkawi, Malaysia. pp. 321–325. doi: 10.1109/istt.2014.7238228
65. Sreenivas GRN. Modern innovations in Poultry Farming. Available online: https://www.srpublication.com/modern-innovations-in-poultry-farming/ (accessed on 26 January 2024).
66. Astill J, Dara RA, Fraser EDG, et al. Smart poultry management: Smart sensors, big data, and the internet of things. Computers and Electronics in Agriculture 2020; 170: 105291. doi: 10.1016/j.compag.2020.105291
67. Lu Y. Industry 4.0: A survey on technologies, applications and open research issues. Journal of Industrial Information Integration 2017, 6: 1–10. doi: 10.1016/j.jii.2017.04.005
68. Mataragas M, Drosinos EH, Tsola E, et al. Integrating statistical process control to monitor and improve carcasses quality in a poultry slaughterhouse implementing a HACCP system. Food Control 2012; 28(2): 205–211. doi: 10.1016/j.foodcont.2012.05.032
69. Manshor N, Abdul Rahiman AR, Yazed MK. IoT-based poultry house monitoring. In: Proceedings of the 2019 2nd International Conference on Communication Engineering and Technology (ICCET); 12–15 April 2019; Nagoya, Japan. pp. 72–75. doi: 10.1109/ICCET.2019.8726880
70. Esteva A, Chou K, Yeung S, et al. Deep learning-enabled medical computer vision. npj Digital Medicine 2021; 4(1). doi: 10.1038/s41746-020-00376-2
71. Fuentes S, Gonzalez Viejo C, Chauhan SS, et al. Non-invasive sheep biometrics obtained by computer vision algorithms and machine learning modeling using integrated visible/infrared thermal cameras. Sensors 2020; 20(21): 6334. doi: 10.3390/s20216334
72. Bhatt C, Hassanien A, Shah NA, Thik J. Barqi breed sheep weight estimation based on neural network with regression. ArXiv 2018. doi: 10.48550/arXiv.1807.10568
73. Cheng M, Yuan H, Wang Q, et al. Application of deep learning in sheep behaviors recognition and influence analysis of training data characteristics on the recognition effect. Computers and Electronics in Agriculture 2022; 198: 107010. doi: 10.1016/j.compag.2022.107010
74. Boesch G. Top applications of computer vision in agriculture (2024 guide) Available online: https://viso.ai/applications/computer-vision-in-agriculture/. https://viso.ai/applications/computer-vision-in-agriculture/ (accessed on 26 January 2024).
75. Sanibel A, Jwade, Guzzomi A, Mian A. On farm automatic sheep breed classification using deep learning. Computers and Electronics in Agriculture 2019; 167: 105055. doi: 10.1016/j.compag.2019.105055
76. Sarwar F, Griffin A, Periasamy P, et al. Detecting and counting sheep with a convolutional neural network. In: Proceedings of the 2018 15th IEEE International Conference on Advanced Video and Signal Based Surveillance (AVSS); 27–30 November 2018; Auckland, New Zealand. pp. 1–6, doi: 10.1109/AVSS.2018.8639306
77. Li Z, Guo R, Li M, et al. A review of computer vision technologies for plant phenotyping. Computers and Electronics in Agriculture 2020; 176: 105672. doi: 10.1016/j.compag.2020.105672
78. Wang YH, Su WH. Convolutional neural networks in computer vision for grain crop phenotyping: A review. Agronomy 2022; 12(11): 2659. doi: 10.3390/agronomy12112659
79. Sankaran S, Khot LR, Espinoza CZ, et al. Low-altitude, high-resolution aerial imaging systems for row and field crop phenotyping: A review. European Journal of Agronomy 2015; 70: 112–123. doi: 10.1016/j.eja.2015.07.004
80. Virlet N, Sabermanesh K, Sadeghi-Tehran P, et al. Field Scanalyzer: An automated robotic field phenotyping platform for detailed crop monitoring. Functional Plant Biology 2017; 44(1): 143. doi: 10.1071/fp16163
81. Frolov K, Fripp J, Nguyen CV, et al. Automated plant and leaf separation: Application in 3D meshes of wheat plants. In: Proceedings of the 2016 International Conference on Digital Image Computing: Techniques and Applications (DICTA); 2016; Gold Coast, QLD, Australia. pp. 1–7, doi: 10.1109/DICTA.2016.7797011
82. Underwood J, Wendel A, Schofield B, et al. Efficient in-field plant phenomics for row-crops with an autonomous ground vehicle. Journal of Field Robotics 2017; 34(6): 1061–1083. doi: 10.1002/rob.21728
83. Bauer A, Bostrom AG, Ball J, et al. Combining computer vision and deep learning to enable ultra-scale aerial phenotyping and precision agriculture: A case study of lettuce production. Horticulture Research 2019; 6(1). doi: 10.1038/s41438-019-0151-5
84. Rebetzke GJ, Jimenez-Berni JA, Bovill WD, et al. High-throughput phenotyping technologies allow accurate selection of stay-green. Journal of Experimental Botany 2016; 67(17): 4919–4924. doi: 10.1093/jxb/erw301
85. Choudhury SD, Goswami S, Bashyam S, et al. Automated stem angle determination for temporal plant phenotyping analysis. In: Proceedings of the 2017 IEEE International Conference on Computer Vision Workshops (ICCVW); 22–29 October 2017; Venice, Italy. pp. 2022–2029, doi: 10.1109/ICCVW.2017.237
86. Mochida K, Koda S, Inoue K, et al. Computer vision-based phenotyping for improvement of plant productivity: A machine learning perspective. GigaScience 2018; 8(1). doi: 10.1093/gigascience/giy153
87. Minervini M, Scharr H, Tsaftaris SA. Image analysis: The new bottleneck in plant phenotyping [Applications corner]. IEEE Signal Processing Magazine 2015; 32(4): 126–131. doi: 10.1109/msp.2015.2405111
88. Toda Y, Okura F. How convolutional neural networks diagnose plant disease. Plant Phenomics 2019; 2019: 1–14. doi: 10.1155/2019/9237136
89. Harandi N, Vandenberghe B, Vankerschaver J, et al. How to make sense of 3D representations for plant phenotyping: A compendium of processing and analysis techniques. Plant Methods 2023; 19: 60. doi: 10.1186/s13007-023-01031-z
90. Fuentes A, Yoon S, Kim S, et al. A robust deep-learning-based detector for real-time tomato plant diseases and pests recognition. Sensors 2017; 17(9): 2022. doi: 10.3390/s17092022
91. Maria F, Efthimios K, Eleni C, et al. Using self organising maps in applied geomorphology. In: Mwasiagi JS (editor). Self Organizing Maps—Applications and Novel Algorithm Design. InTech; 2011. doi: 10.5772/13265
92. Tataridas A, Kanatas P, Chatzigeorgiou A, et al. Sustainable crop and weed management in the era of the EU green deal: A survival guide. Agronomy 2022; 12(3): 589. doi: 10.3390/agronomy12030589
93. Pantazi XE, Tamouridou AA, Alexandridis TK, et al. Evaluation of hierarchical self-organising maps for weed mapping using UAS multispectral imagery. Computers and Electronics in Agriculture 2017; 139: 224–230. doi: 10.1016/j.compag.2017.05.026
94. Zhai Z, Martínez Ortega JF, Lucas Martínez N, et al. A mission planning approach for precision farming systems based on multi-objective optimization. Sensors 2018; 18(6): 1795. doi: 10.3390/s18061795
95. Zhang R, Wang C, Hu X, et al. Weed location and recognition based on UAV imaging and deep learning. International Journal of Precision Agricultural Aviation 2018; 1(1): 23–29. doi: 10.33440/j.ijpaa.20200301.63
96. Hasan ASMM, Sohel F, Diepeveen D, et al. A survey of deep learning techniques for weed detection from images. Computers and Electronics in Agriculture 2021; 184: 106067. doi: 10.1016/j.compag.2021.106067
97. Nayak A. The economics of applications of artificial intelligence and machine learning in agriculture. International Journal of Pure & Applied Bioscience 2019; 7(1): 296–305. doi: 10.18782/2320-7051.7324
98. Farooq O, Gill J. Vegetable grading and sorting using artificial intelligence. International Journal for Research in Applied Science and Engineering Technology 2022; 10(3): 13–21. doi: 10.22214/ijraset.2022.40407
99. Pathak AK, Kumar M, Sharma SK. Grading and sorting of fruits and vegetables for increasing farmer’s income. Available online: https://krishijagran.com/featured/grading-and-sorting-of-fruits-and-vegetables-for-increasing-farmer-s-income/ (accessed on 26 January 2024).
100. Llobet E, Hines EL, Gardner JW, et al. Non-destructive banana ripeness determination using a neural network-based electronic nose. Measurement Science and Technology 1999; 10(6): 538–548. doi: 10.1088/0957-0233/10/6/320
101. Bennedsen BS, Peterson DL, and Tabb A. Identifying apple surface defects using principal components analysis and artificial neural networks. Transactions of American Society of Agricultural and Biological Engineers 2007; 50(6): 2257–2265. doi: 10.13031/2013.24078
102. Zakaria A, Shakaff AYM, Masnan MJ, et al. Improved maturity and ripeness classifications of Magnifera Indica cv. Harumanis mangoes through sensor fusion of an electronic nose and acoustic sensor. Sensors 2012; 12(5): 6023–6048. doi: 10.3390/s120506023
103. Nur BAM, Ahmed SK, Ali Z, et al. Agricultural produce sorting and grading using support vector machines and fuzzy logic. In: Proceedings of the 2009 IEEE International Conference on Signal and Image Processing Applications; 18–19 November 2009; Kuala Lumpur, Malaysia. pp. 391–396 doi: 10.1109/icsipa.2009.5478684
104. Yosef, Al Ohali. Computer vision-based date fruit grading system: Design and implementation. Journal of King Saud University—Computer and Information Sciences 2011; 23(1): 29–36. doi: 10.1016/j.jksuci.2010.03.003
105. Nandi CS, Tudu B, Koley C. An automated machine vision-based system for fruit sorting and grading. In: Proceedings of the 2012 Sixth International Conference on Sensing Technology (ICST); 18–21 December 2012; Kolkata, India. pp. 195–200. doi: 10.1109/icsenst.2012.6461669
106. Hadha A, Faris M, Utomo PG, et al. Portable smart sorting and grading machine for fruits using computer vision. In: Proceedings of the 2013 International Conference on Computer, Control, Informatics and Its Applications (IC3INA); 19–21 November 2013; Jakarta, Indonesia; pp. 71–75. doi: 10.1109/IC3INA.2013.6819151
107. Intellias. AI in agriculture—The future of farming. Available online: https://intellias.com/artificial-intelligence-in-agriculture/ (accessed on 26 January 2024).
108. Paymode AS, Mohite JN, Shinde UB, Malode VB. Artificial intelligence for agriculture: A technique of vegetables crop onion sorting and grading using deep learning. International Journal Of Advance Scientific Research and Engineering Trends 2021; 6(4). doi: 10.51319/2456-0774.2021.4.0004
109. Patil PU, Lande SB, Nagalkar VJ, et al. Grading and sorting technique of dragon fruits using machine learning algorithms. Journal of Agriculture and Food Research 2021; 4: 100118. doi: 10.1016/j.jafr.2021.100118
110. Mushiri T, Tende L. Automated grading of tomatoes using artificial intelligence: The case of Zimbabwe. In: Strydom M, Buckley S (editors). AI and Big Data’s Potential for Disruptive Innovation. IGI Global; 2020. pp. 216–239. doi: 10.4018/978-1-5225-9687-5.ch008
111. Everaerts J. The use of unmanned aerial vehicles (UAVs) for remote sensing and mapping. The International Archives of the Photogrammetry, Remote Sensing and Spatial Information Sciences 2008; XXXVII: Part B1.
112. Colomina I, Molina P. Unmanned aerial systems for photogrammetry and remote sensing: A review. ISPRS Journal of Photogrammetry and Remote Sensing 2014; 92: 79–97. doi: 10.1016/j.isprsjprs.2014.02.013
113. Bendig J, Bolten A, Bareth G. Introducing a low-cost mini-UAV for thermal—And multispectral-imaging. The International Archives of the Photogrammetry, Remote Sensing and Spatial Information Sciences 2012; XXXIX-B1: 345–349. doi: 10.5194/isprsarchives-xxxix-b1-345-2012
114. Primicerio J, Di Gennaro SF, Fiorillo E, et al. A flexible unmanned aerial vehicle for precision agriculture. Precision Agriculture 2012; 13(4): 517–523. doi: 10.1007/s11119-012-9257-6
115. Garre P, Harish A. Autonomous agricultural pesticide spraying UAV. IOP Conference Series: Materials Science and Engineering 2018; 455: 012030. doi: 10.1088/1757-899X/455/1/0120
116. Malveaux C, Hall SG, Price R. Using drones in agriculture: unmanned aerial systems for agricultural remote sensing applications. American Society of Agricultural and Biological Engineers 2014. doi: 10.13031/aim.20141911016
117. Hogan SD, Kelly M, Stark B, et al. Unmanned aerial systems for agriculture and natural resources. California Agriculture 2017; 71(1): 5–14. doi: 10.3733/ca.2017a0002
118. El Bilali H, Allahyari MS. Transition towards sustainability in agriculture and food systems: Role of information and communication technologies. Information Processing in Agriculture 2018; 5(4): 456–464. doi: 10.1016/j.inpa.2018.06.006
119. Mogili UM, Deepak BBVL. Review on application of drone systems in precision agriculture. Procedia Computer Science 2018; 133: 502–509. doi: 10.1016/j.procs.2018.07.063
120. Veroustraete F. The rise of the drones in agriculture. EC Agriculture 2.2 2015; 325–327.
121. Puri V, Nayyar A, Raja L. Agriculture drones: A modern breakthrough in precision agriculture. Journal of Statistics and Management Systems 2017; 20(4): 507–518. doi: 10.1080/09720510.2017.1395171
122. Ahirwar S, Swarnkar R, Bhukya S, et al. Application of drone in agriculture. International Journal of Current Microbiology and Applied Sciences 2019; 8(01): 2500–2505. doi: 10.20546/ijcmas.2019.801.264
123. Ayamga M, Tekinerdogan B, Kassahun A. Exploring the challenges posed by regulations for the use of drones in agriculture in the African context. Land 2021; 10(2): 164. doi: 10.3390/land10020164
124. Ren Q, Zhang R, Cai W, et al. Application and development of new drones in agriculture. IOP Conference Series: Earth and Environmental Science 2020; 440(5): 052041. doi: 10.1088/1755-1315/440/5/052041
125. Garnot VSF, Landrieu L. Lightweight temporal self-attention for classifying satellite images time series. In: Proceedings of the International Workshop on Advanced Analytics and Learning on Temporal Data; 18 September 2020; Ghent, Belgium. pp. 171–181. doi: 10.1007/978-3-030-65742-0_12
126. Schneider M, Korner M. Harnessing administrative data inventories to create a reliable transnational reference database for crop type monitoring. In: Proceedings of the IGARSS 2022–2022 IEEE International Geoscience and Remote Sensing Symposium; 17–22 July 2022; Kuala Lumpur, Malaysia. pp. 5385–5388. doi: 10.1109/IGARSS46834.2022.9883089
127. Garnot VSF, Landrieu L. Panoptic segmentation of satellite image time series with convolutional temporal attention networks. In: Proceedings of the IEEE/CVF International Conference on Computer Vision (ICCV); 10–17 October 2021; Montreal, QC, Canada. pp. 4872–4881. doi: 10.48550/arXiv.2107.07933
128. Quinton F, Landrieu L. Crop rotation modeling for deep learning-based parcel classification from satellite time series. Remote Sensing 2021; 13(22): 4599. doi: 10.3390/rs13224599
129. Liu Y, Zhao W, Chen S, et al. Mapping crop rotation by using deeply synergistic optical and SAR time series. Remote Sensing 2021; 13(20): 4160. doi: 10.3390/rs13204160
130. Shiraly K. How computer vision in agriculture is boosting productivity and yields. Available online: https://www.width.ai/post/computer-vision-in-agriculture (accessed on 26 January 2024).
131. Stanhope TP, Adamchuk VI, Roux JD. Computer vision guidance of field cultivation for organic row crop production. American Society of Agricultural and Biological Engineers Annual International Meeting 2014. doi: 10.13031/aim.20141909498
132. Braeger S, Foroosh H. Curvature augmented deep learning for 3D object recognition. In: Proceedings of the 2018 25th IEEE International Conference on Image Processing (ICIP); 7–10 October 2018; Athens, Greece. pp. 3648–3652. doi: 10.1109/ICIP.2018.8451487
133. Cué La Rosa LE, Queiroz Feitosa R, Nigri Happ P, et al. Combining deep learning and prior knowledge for crop mapping in tropical regions from multitemporal SAR image sequences. Remote Sensing 2019; 11(17): 2029. doi: 10.3390/rs11172029
134. Niall OM, Sean C, Anderson C, et al. Deep learning vs. traditional computer vision. In: Arai K, Kapoor S (editors). Advances in Computer Vision. CVC 2019. Advances in Intelligent Systems and Computing. Springer, Cham; 2020. doi: 10.1007/978-3-030-17795-9_10
135. Syal A, Garg D, Sharma S. A survey of computer vision methods for counting fruits and yield prediction. International Journal of Computer Science Engineering (IJCSE) 2013; 2(6).
136. Boatswain Jacques AA, Adamchuk VI, Park J, et al. Towards a machine vision-based yield monitor for the counting and quality mapping of shallots. Frontiers in Robotics and AI 2021; 8. doi: 10.3389/frobt.2021.627067
137. Payne A, Walsh KB. Machine vision in estimation of fruit crop yield. In: Plant Image Analysis: Fundamentals and Applications. CRC Press; 2013. doi: 10.1201/b17441-17
138. Raphael L, Cohen O, Naor A. Determination of the number of green apples in RGB images recorded in orchards. Computers and Electronics in Agriculture 2012; 81: 45–57. doi: 10.1016/j.compag.2011.11.007
139. Pandit RB, Tang J, Liu F, et al. Development of a novel approach to determine heating pattern using computer vision and chemical marker (M-2) yield. Journal of Food Engineering 2007; 78(2): 522–528. doi: 10.1016/j.jfoodeng.2005.10.039
140. Patel HN, Jain RK, Joshi MV. Automatic segmentation and yield measurement of fruit using shape analysis. International Journal of Computer Applications 2012; 45(7): 19–24.
141. Yoosefzadeh-Najafabadi M, Earl HJ, Tulpan D, et al. Application of machine learning algorithms in plant breeding: Predicting yield from hyperspectral reflectance in soybean. Frontiers in Plant Science 2021; 11. doi: 10.3389/fpls.2020.624273
142. Kapach K, Barnea E, Mairon R, et al. Computer vision for fruit harvesting robots—State of the art and challenges ahead. International Journal of Computational Vision and Robotics 2012; 3(1/2): 4. doi: 10.1504/ijcvr.2012.046419
143. Chlingaryan A, Sukkarieh S, Whelan B. Machine learning approaches for crop yield prediction and nitrogen status estimation in precision agriculture: A review. Computers and Electronics in Agriculture 2018; 151: 61–69. doi: 10.1016/j.compag.2018.05.012
144. Kakani V, Nguyen VH, Kumar BP, et al. A critical review on computer vision and artificial intelligence in food industry. Journal of Agriculture and Food Research 2020; 2: 100033. doi: 10.1016/j.jafr.2020.100033
145. Boesch G. Top applications of computer vision in agriculture (2024 guide) Available online: https://viso.ai/applications/computer-vision-in-agriculture/. https://viso.ai/applications/computer-vision-in-agriculture/ (accessed on 26 January 2024).
146. Ahmmed P, Reynolds J, Hamada S, et al. Novel 3D-printed electrodes for implantable biopotential monitoring. In: Proceedings of the 2021 43rd Annual International Conference of the IEEE Engineering in Medicine & Biology Society (EMBC); 1–5 November 2021; Mexico. pp. 7120–7123. doi: 10.1109/EMBC46164.2021.9630055
147. Wang Y, Mücher S, Wang W, et al. A review of three-dimensional computer vision used in precision livestock farming for cattle growth management. Computers and Electronics in Agriculture 2023; 206: 107687. doi: 10.1016/j.compag.2023.107687
(126 Abstract Views, 9 PDF Downloads)
Refbacks
- There are currently no refbacks.
Copyright (c) 2024 Bojana Petrovic, Vesna Tunguz, Petr Bartos

This work is licensed under a Creative Commons Attribution-NonCommercial 4.0 International License.
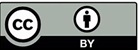
This site is licensed under a Creative Commons Attribution 4.0 International License.