
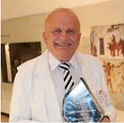
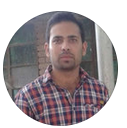
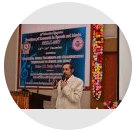
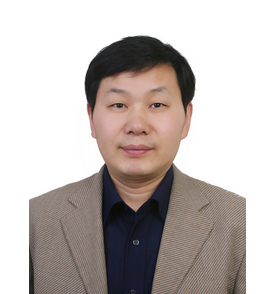
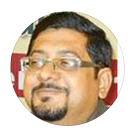
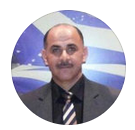
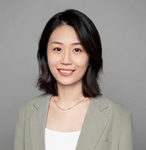


Section Collection Information
Dear Colleague,
Statistics and Probability section work focused on all aspects of mathematics that employs the use of probabilistic or statistical methods: theory, application, computation, exploitation, and more. The section welcomes submissions from researchers who seek to advance fundamentals of probability and statistics, as well as to develop applications of these principles within other related disciplines.The fields are applied to Biology,Data science,Engineering and Computer Science,Economics,Geographic information systems,Medicine and Other potential areas. Areas covered by this section include, but are not limited to some new statistical theory for contemporary methods ,new analytical tools to establish new statistical and probability theory and statistical and probabilistic methods in interesting application domains and other topics related to the statistics and probability community.
An important problem in the inferential statistics is to estimate the finite population cumulative distribution function (CDF), which arises when the interest is to determine the proportion of values of a study variable that are less than or equal to a certain value. In survey sampling, there are several sampling schemes that may be used to develop an estimator of the finite population CDF, namely the simple random sampling (SRS), ranked-set sampling (RSS), stratified random sampling, cluster sampling (CS), to name a few.
A common approach in survey sampling is to increase the precision of an estimator with suitable use of auxiliary information.The ratio, regression and product-type estimators are prime examples as these estimators require supplementary information on one or more auxiliary variables along with the information on a study variable to increase their relative efficiencies.A significant amount of research work has been done in the literature of survey sampling to develop new improved estimators of the population parameters, which include the population mean, total, CDF, median, etc.So, one can estimate the finite population parameters, that is, total, mean, CDF and variance in a complex survey sampling with or without using the auxiliary information.There is a lot of work have to do in this space.
In recent years machine learning techniques are widely used for analyzing medical data. Machine learning algorithms differentiate the healthy person to the patients. For prediction classification and diagnosis of diseases different machine learning techniques such as artificial neural network are to be used.From machine learning technique one can detect and predict the disease easily and accurately with low price.
This section provides an Open-Access publishing forum for the applied probability and statistics community and also encourages interdisciplinary collaboration through the development of articles.We look forward to receiving your contributions.
Prof. Dr. Mohsin Abbas
Prof. Dr. Len Gelman
Prof. Dr. Intekhab Alam
Prof. Dr. Soubhik Chakraborty
Prof. Dr. Wanyang Dai
Prof. Dr. Sanjib Kumar Datta
Prof. Dr. Reda Abdelftah shenawy
Prof. Dr. Wenzhe Xu
Prof. Dr. Haibin Hang
Prof. Dr. Muhammad Mujtaba Shaikh
Section Editors