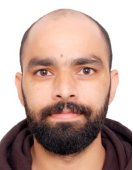
Section Collection Information
Dear colleagues,
Image Time Series Analysis is a field of study that aligns closely with my research interests in machine learning, deep learning, and remote sensing. In this context, it involves the systematic exploration of time-varying remotely sensed images, which play a critical role in addressing various real-world challenges. These challenges range from monitoring climate change and assessing agricultural applications, to managing and conserving natural resources. Image Time Series Analysis is an essential and dynamic field within remote sensing and computer science. This specialized section within Remote Sensing Technology (RST) focuses on the exploration, interpretation, and exploitation of time-varying remotely sensed images. It plays a crucial role in understanding and addressing a wide range of complex environmental and societal challenges.
Key Focus Areas:
i. Monitoring Environmental Changes: This section emphasizes the utilization of image time series to monitor and analyze environmental changes, including land-use transformations, deforestation, urban growth, and the dynamics of natural ecosystems. Researchers delve into the intricacies of Earth's evolution over time, helping us better comprehend long-term environmental trends.
ii. Climate Change Analysis: A significant aspect of Image Time Series Analysis is the examination of climate change effects. Researchers explore how climate change impacts are documented through remotely sensed imagery, enabling the development of critical insights into global warming, glacial retreat, and the shifting climate patterns that affect our planet.
iii. Agricultural Applications: The section also delves into the agricultural domain, where the analysis of time series data helps optimize crop management, assess the health of crops, and enhance food security. This research area fosters innovations in precision agriculture, remote crop monitoring, and the sustainable use of farmland.
Methodological Approaches:
Researchers in this section employ a diverse set of methodologies, including machine learning, deep learning, image processing, and data analytics, to extract meaningful information from image time series data. They develop cutting-edge algorithms and models for change detection, feature extraction, and predictive modeling to provide valuable insights.
Interdisciplinary Collaboration:
Image Time Series Analysis thrives on interdisciplinary collaboration, as it bridges the gap between the worlds of remote sensing, computer science, and environmental science. This section encourages researchers from various backgrounds to unite their expertise to tackle complex challenges, enabling a holistic approach to understanding temporal changes.
Global Relevance:
The section recognizes the global relevance of its research, as the findings contribute to addressing some of the most pressing issues of our time. It plays a significant role in advancing climate science, sustainable agriculture, disaster management, and environmental policy.
Image Time Series Analysis section is at the forefront of harnessing the power of time-varying remotely sensed images. It serves as a platform for researchers to exchange knowledge, advance methodologies, and apply their expertise to solve real-world challenges in environmental monitoring, climate change analysis, and agricultural innovation. This section is dedicated to disseminating valuable insights that can drive positive change on a global scale.
Dr. Amit Sharma
Section Editors