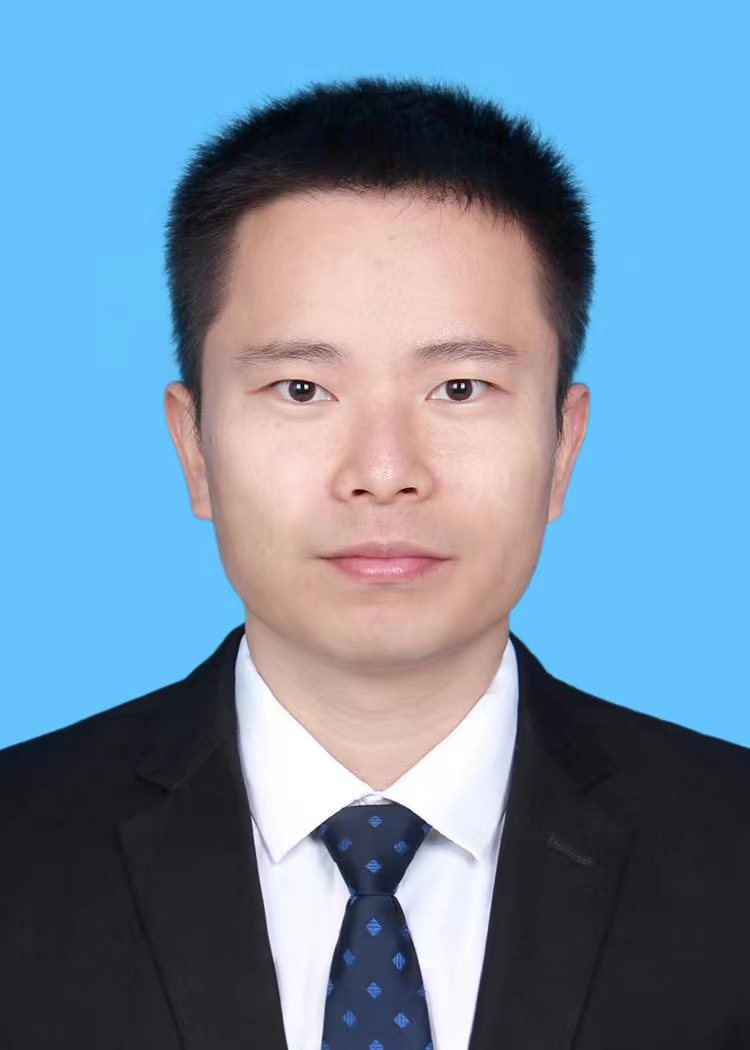
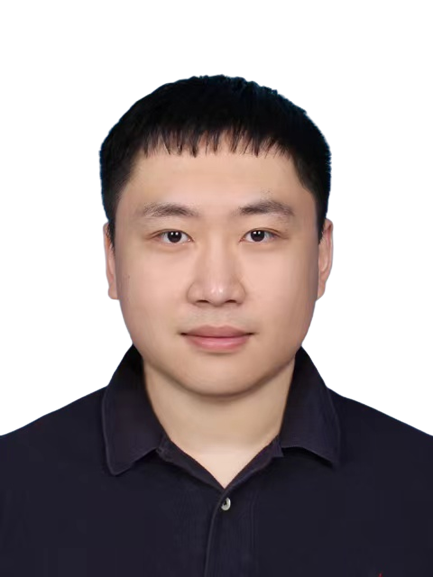
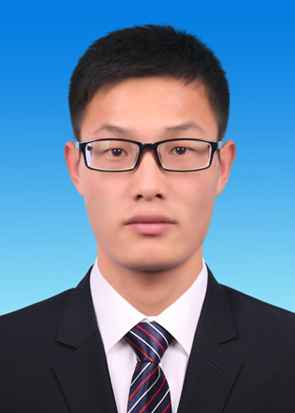
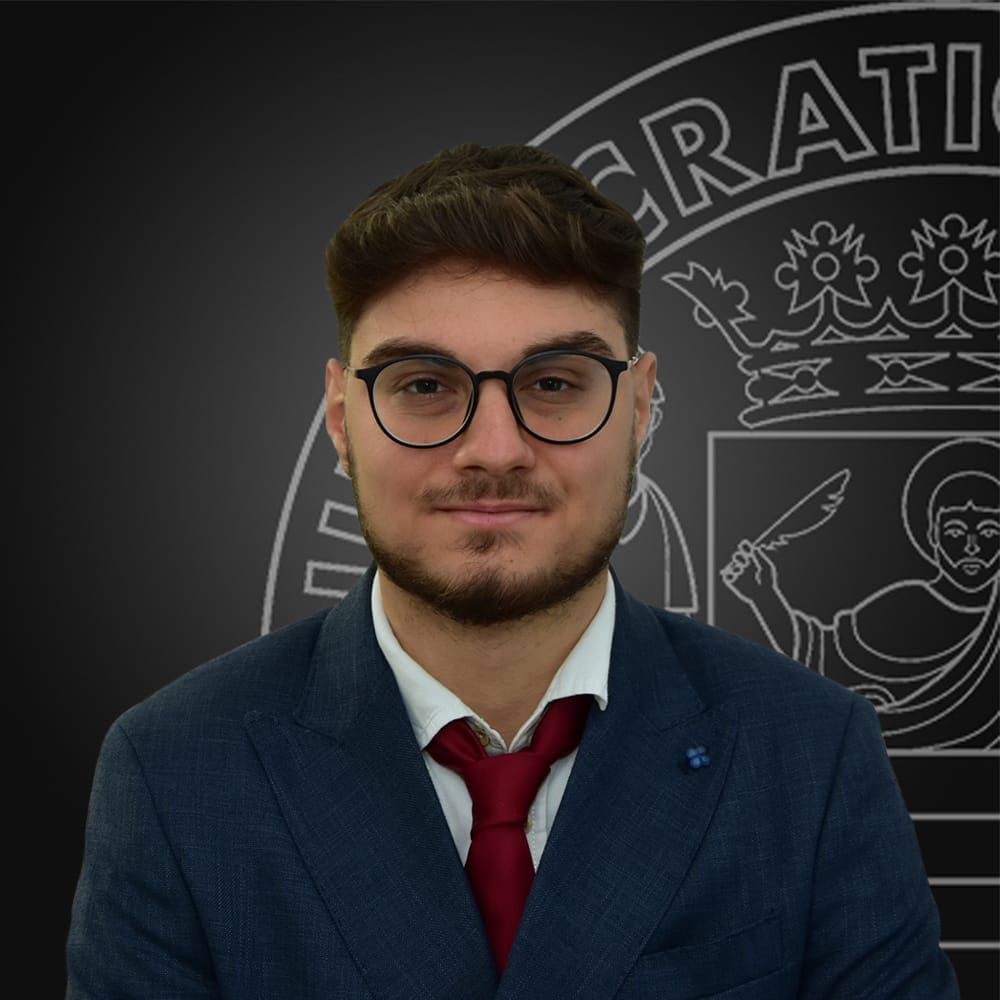
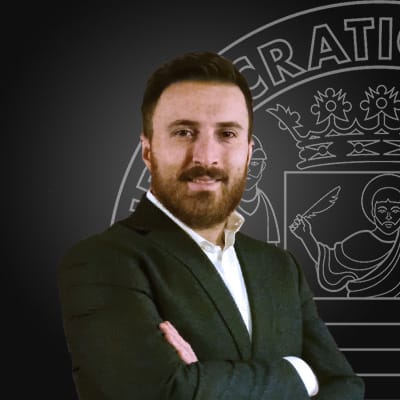
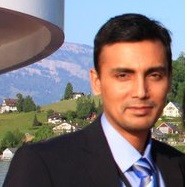
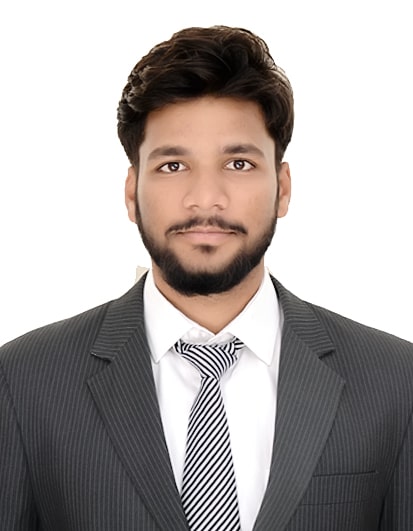
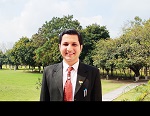
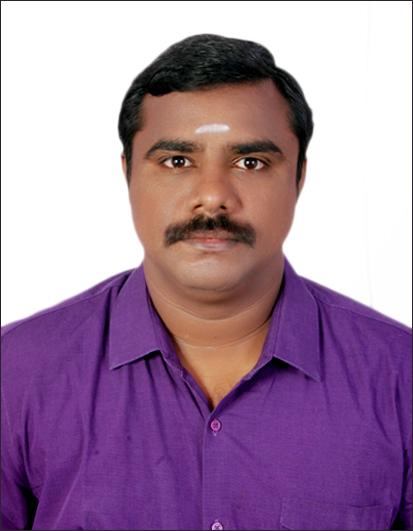
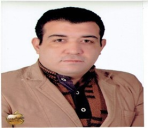
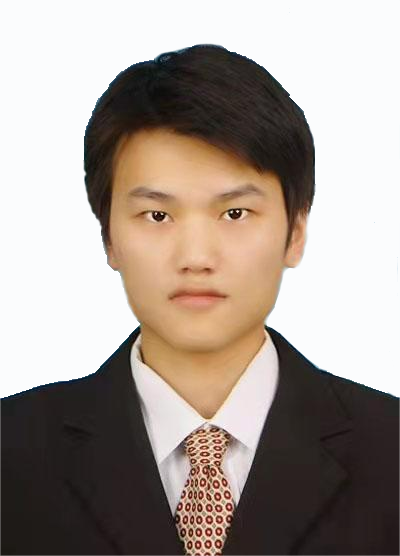
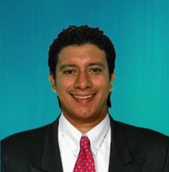
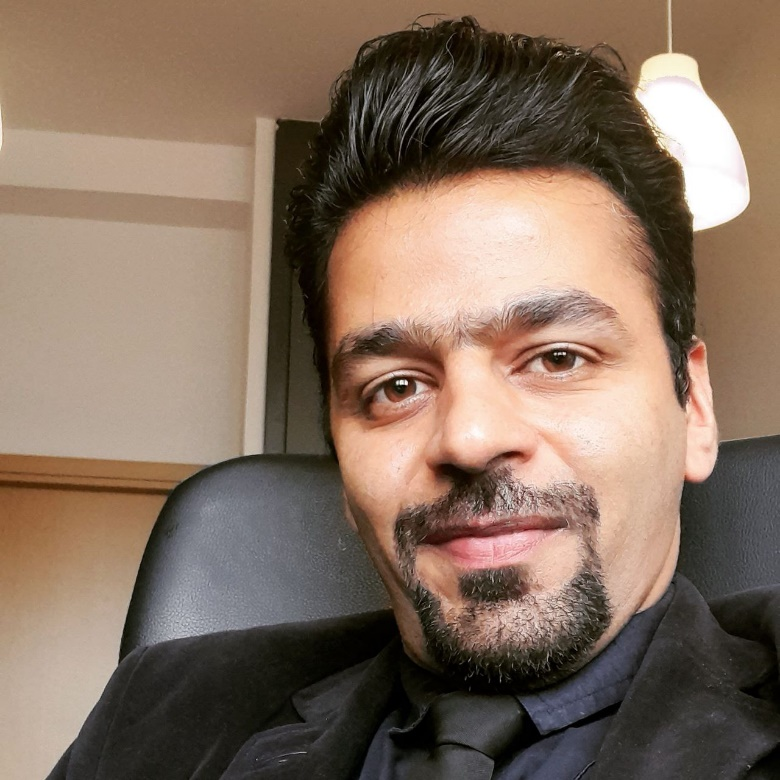
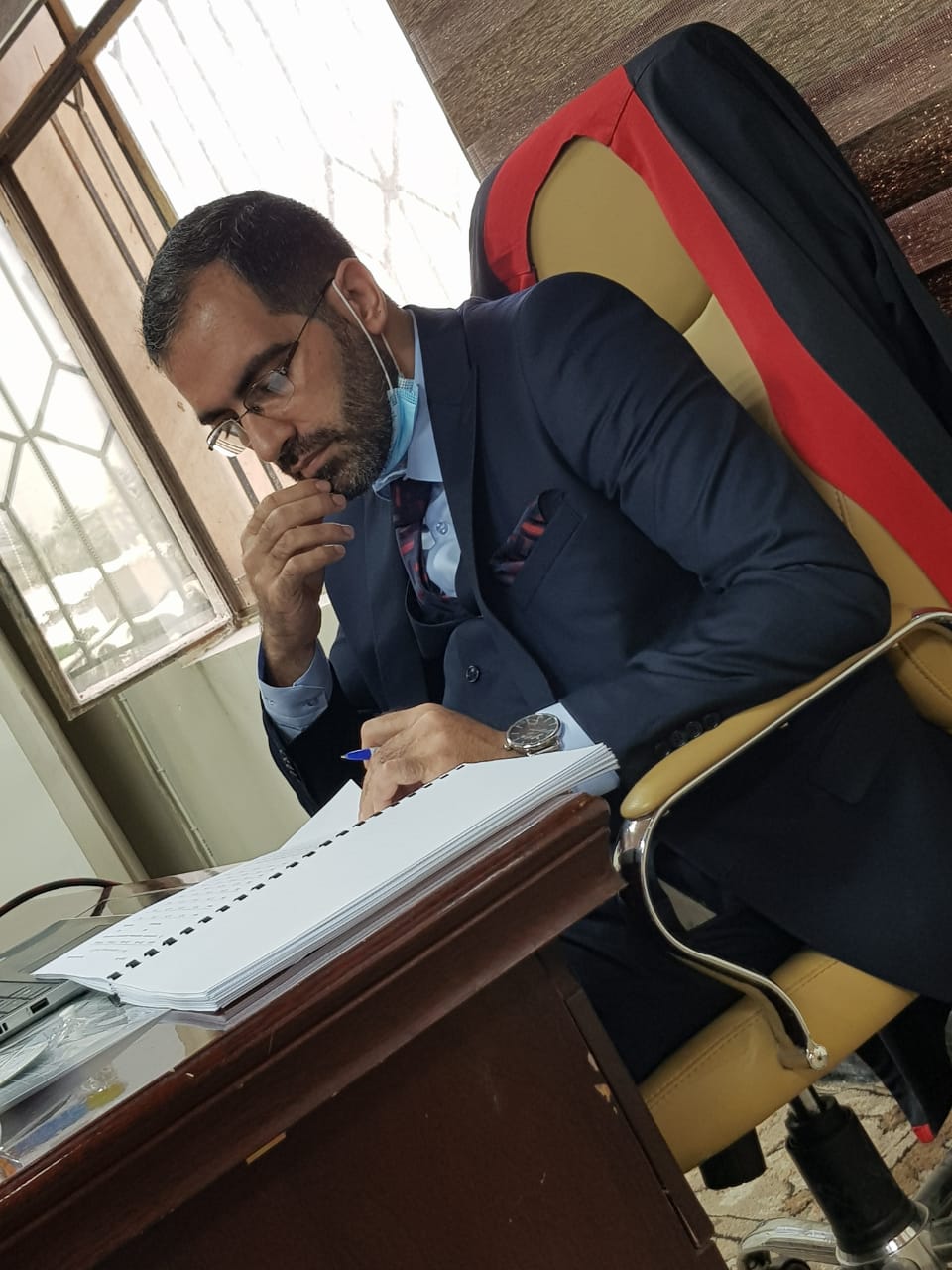
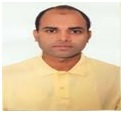
Section Collection Information
Dear Colleagues,
In recent years, the concept of Artificial Intelligence (AI) and Systems Control have been increasingly penetrated to industrial sectors, encompassing smart grid, transportation, manufacturing, robot, and beyond, aiming at revolutionizing operation paradigm and raising efficiency. The incorporation of AI-based methodologies, such as deep learning, reinforcement learning, and neural networks, within the realm of systems control has engendered a profound shift, unveiling novel prospects for the refinement of intricate operational processes, the augmentation of decision-making capabilities, and the amelioration of overall system efficacy. In an endeavor to strengthen the understanding of AI-based control methodologies and techniques for modern industrial applications, comprehensively and profoundly exploring the latest developments, challenges, and opportunities in this interdisciplinary domain from both theory and application perspectives is of urgency.
Therefore, we are interested in the exploring the interactions between the system and environment, as well as the internal dynamics of the complex system. These investigations serve as the foundational underpinnings upon which the formulation of sophisticated AI-driven control strategies is predicated, and subsequently, they facilitate the critical appraisal of the viability and effectiveness of these strategies within specific application domains.
To achieve this, the methods beyond the state-of-the-art ones are valuable to be envisaged and proposed considering their benefits to a broad range of practical applications. Both research articles and literature reviews within the scope of AI and Systems Control are welcome. We look forward to receiving your contributions.
Dr. Zhijian Hu
Assistant Professor Renjie Ma
Dr. Ruiping Wang
Section Editors